Datasets
This study was approved by the Institutional Review Board of Yonsei University Dental Hospital (IRB No. 2-2021-0024). De-identified participant data were used in this retrospective study and therefore, the written consent requirement was waived. This study was performed in accordance with the Declaration of Helsinki. The criteria for selection were panoramic images of patients diagnosed with sialolith and lymph node calcification after visiting the Department of Advanced General Dentistry and Department of Oral and Maxillofacial Surgery at Yonsei University Dental Hospital from June 2006 to November 2020. Moreover, among the patients admitted to the departments of Cardiovascular Surgery or Cardiology of Yonsei University Severance Hospital and requested collaboration with the Department of Integrated Dentistry at Yonsei University Dental Hospital from June 2006 to November 2020, those diagnosed with carotid artery calcification on panoramic images were used.
Among 163 patients diagnosed with sialolith, patients with panoramic radiographs were primarily screened. Afterwards, 60 patients were randomly selected to be used for AI testing. There were 26 patients who were diagnosed with lymph node calcification and took panoramic radiographs were all selected. For carotid artery calcification, 3928 patients with panoramic radiographs were first screened, and then 60 of them were randomly selected.
Regarding the exclusion criteria, cases with only a preliminary/presumed diagnosis from the medical record or panoramic image but without a definitive diagnosis were excluded. Cases with medical records but no panoramic images, or when soft tissue calcification was not clearly observed in the study, were also excluded (Table 1).
Fast region-based convergence neural network system
In this study, rather than complex processes and pretreatments, a fast region-based convergence neural network (FAST-RCNN) with ResNet Backbone that detected suspected sialolith, lymph node calcification, and carotid artery calcification was used to assist GDs as well as OMRs in diagnosing the three diseases through panoramic images. ResNet improves the accuracy by reducing the depth of the learning layer and increasing the performance compared of the convolutional neural network (CNN) model, which is an existing image analysis model, through residual learning. Therefore, Fast-RCNN divides the characteristics of objects in sialolith, lymph node calcification, and carotid artery calcification, into CNN-based feature maps with different characteristics and then trains them through a CNN. Subsequently, the feature map from the CNN passes through a region proposal network that evaluates the degree of detection judgment17, receives the classification and interval values of the object, and resizes the box to be placed in the fully connected (FC) layer using ROI pooling.
Fast-RCNN is a method that derives better accuracy than existing object detection algorithms by extracting image features and minimizing noise for image analysis. Fast-RCNN is composed of a convolution feature map and ROI feature vector. The convolution feature map delivers images to the convolution and max-pooling layers, and the received information is placed as features in the ROI feature vector map. Now, we can apply classification and bounding box regression to this vector to obtain each loss, and train the entire model by back propagating it. At this time, it is necessary to properly weave the classification loss and the bounding box regression, which is called a multi-task loss. The formula is as follows (1). First, as an input, p is the probability value of K + 1 (K objects + 1 background, class representing no object) obtained through soft max. where u is the ground truth label value of the ROI. Next, we apply bounding box regression to the result, which returns tk values that adjust the x, y, w, and h values for K + 1 classes, respectively. In the loss function, only the value corresponding to the ground truth label among these values is fetched, which corresponds to tu. The v corresponds to the ground truth bounding box adjustment value.
$$begin{array}{*{20}l} {text{L}}left( {{text{p}},{text{u}},{text{t}}^{{text{u}}} ,{text{v}}} right) = L_{cls} left( {p,u} right) + lambda left[ {u ge 1} right]L_{loc} left( {t^{u} , v} right), hfill \ {text{p}} = left( {{text{p}}_{0} , ldots ,{text{ p}}_{{text{K}}} } right). hfill \ end{array}$$
(1)
Then, it receives the bounding box regression prediction value corresponding to the correct answer label and the ground truth adjustment value. For each of x, y, w, and h, the difference between the predicted value and the label value is calculated, and the sum passed through a function called smoothL1 is calculated. As a result, the prediction process is completed as (2).
$${text{Smooth}}_{text{L}1}left(xright)= left{begin{array}{ll}0.5{x}^{2}& text{if }left|xright|<1\ left|xright|-0.5& otherwiseend{array}.right.$$
(2)
This model has a simpler pre-processing and learning process than an algorithm that segments the entire detailed area; additionally, it extracts image features and minimizes noise. Therefore, it showcases a higher accuracy than conventional CNNs18. As shown in Fig. 1 Fast-RCNN consists of a convolution feature map (CNN-based) and a region of interest (ROI) derived from propositional feature vectors. The convolution feature map extracts features of an entire image using convolution and max-pooling layers, generates vector values for these features, and delivers them to the ROI pooling layer19. Subsequently, the ROI feature vector sets the various ranges of spaces for the features of the received image and converts these features into a map. The converted maps are then moved to the fully connected FC layers. The final image class is determined by calculating the probability for one of the K object classes and then evaluating the same of each set for the K classes. The model was trained for 100,000 epochs, and the learning rate was set from 0.001 through 0.000001. For actual learning, Tensorflow object detection library was used, and training was performed until reaching the maximum step with height and wide strides of 16 for 4 classes.
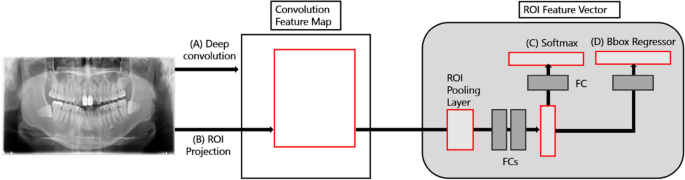
Fast RCNN network model for predicting soft tissue calcification. (A) Deep convolution suggested candidate. (B) Candidate boxes are executed in Pooling Layer. (C) Softmax determines class name of disease. (D) Regressor estimates probability index for each ROI Layer.
The Fast-RCNN divides the characteristics of objects in sialolith, lymph node calcification, and carotid artery calcification into CNN-based feature maps with different characteristics and then trains them through the CNN. Subsequently, the feature map from the CNN passes through a region proposal network that evaluates the degree of detection judgment17, receives the classification and interval values of the object, and resizes the box to be placed in the FC layer using ROI pooling. This model determined diseases by dividing the cases into a total of four classes, namely sialolith, lymph node calcification, carotid artery calcification, and normal, using the output and loss of values that passed the FC layer to find the optimal category for the object.
Observer study
All observers assessed the presence or absence of sialolith, lymph node calcification, and carotid artery calcification in panoramic images over two sessions on different days, distinguishing left and right (Fig. 2).
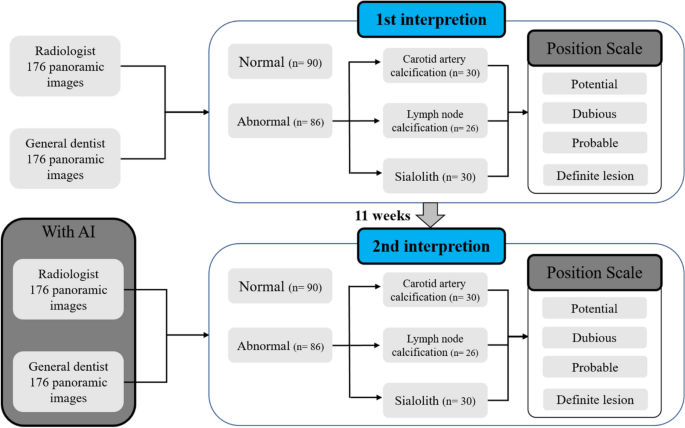
Schematic of observer study design. The first readings were 176 images without artificial intelligence. Abnormal images are separated first and classified as carotid artery calcification, lymph node calcification, or sialolith. Reading confidence is then expressed on a 1 through 4 confidence scale. The second reading takes place 11 weeks after the first reading, and 176 images, different from the first reading, are read using the AI algorithm. Normal and abnormal images are classified first and then a confidence scale of 1 through 4 is displayed.
In the first reading without AI, each participant watched 176 panoramic images and classified them as normal or abnormal without the help of AI, and if an image was deemed abnormal, they reported the possibility of sialolith, lymph node calcification, and carotid artery calcification on a confidence scale (1–4 points) for left and right side. In the confidence scale, the numbers one, two, three, and four meant potential, dubious, probable, and definite lesions, respectively.
The second reading with AI was conducted 11 weeks after the first reading session. As the panoramic images diagnosed with lymph node calcification were the same as those in the first reading, secondary readings were performed at a sufficient interval to erase the memories of the images. The same observers read under identical conditions, referring to the diagnostic results of the developed AI.
Desktop monitors (HP P24h G4 FHD, screen resolution 1920 × 1080 pixels) were used for reading, and the reading environment around the monitor was the same for both sessions. The time required for the first and second readings of all 176 images together was measured in seconds; no upper limit was specified.
Data analysis
For each observer, the time required for the first and second readings was compared and evaluated. For each panoramic image, the actual diagnosis and the observer’s diagnosis were compared for both left and right sides—one point was scored if both sides were correct, and zero points if either side was wrong. The perfect score was 176 points per person during each session, which was converted to a scale of 0 to 100 points.
Additionally, the sensitivity and specificity of the observers’ first and second readings were compared and evaluated, and the receiver operating characteristic (ROC) was calculated using scale values. Finally, the accuracy of the AI algorithm was compared and evaluated using the sensitivity and specificity results of the readings.