Quality of generated oral images
In this study, we present synthetic intraoral images using PGGAN. When the dentists evaluated the visual quality of the generated images, only the resolution of 1024 × 1024 showed a significantly higher d prime (Fig. 3). Since 1024 × 1024 is a higher resolution, the generated image may have been noticeably rougher than the other images, which led to easy discrimination by the dentists. This is considered to be consistent with the fact that the SWD value of 1024 × 1024 is only higher than that of the other resolutions (Table 5). There is a possibility that artifacts in the generated images may be invisibly small and hidden at low resolutions. However, even if a quantitative metric such as SWD provides the best results, not all generated images can be used for education. It is desirable to use the generated images after filtering by experts, so both quantitative and qualitative evaluations were performed in our study. If the pediatric dentists cannot recognize the artifacts, the generated images are considered to be acceptable for educational materials. In other words, it is considered that the generated intraoral images with lower resolutions are so good that the dentist cannot distinguish whether they are real or generated images.
The reason why the SWD value of 1024 × 1024 has been increased by data augmentation is considered to be the filled pixels on boundaries. Although the quality of the image deteriorates owing to the filled pixels of boundaries, the filled area is small at 512 × 512 or lower resolutions, and it is considered that the increase in image variation by data augmentation contributes to a decrease in the SWD value. On the other hand, at 1024 × 1024 resolution, because the filled area is larger than that of lower resolutions, it is considered that the influence of deterioration of image quality is more affected than the increase in image variation, which leads to an increase in the SWD value. At 512 × 512 or lower resolutions, data augmentation decreased the SWD values. If the filled pixels of the image boundaries were cut out, the remaining central images could be of better quality than the images generated by PGGAN trained without dataaugmentation.
Focusing on the teeth is one of the key factors in distinguishing whether an image is real or generated (Fig. 4). Some teeth in generated images look strange compared with natural teeth; however, teeth alignment or soft tissue, such as the tongue, lips, and nose, look realistic enough (Fig. 5). This is because, for primary and permanent teeth, there are many types of anatomical shapes, colors, fissures, cusps, and outlines than types of alignments or soft tissues for each person; hence, PGGAN cannot learn and generate tooth features with our limited dataset. So people who use the generated images need to examine whether the images are misleading. Our results indicate that the teeth were critical for detecting the generated images. However, this does not mean that other factors are not important. It should be noted that alignment, soft tissue, or any other intraoral information is equally important in both dental education and dental treatment.
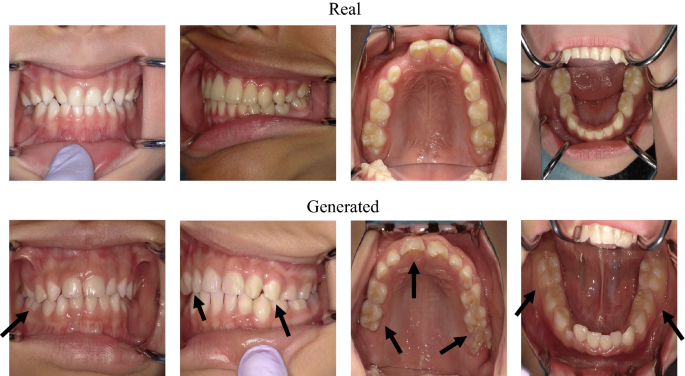
Comparison between real and generated intraoral images. Arrows indicate points of strange teeth. Tooth alignment and soft tissues in both images are similar.
Research limitation
The efficiency of generating realistic images using PGGAN markedly depends on the number of learned images. Even if dentists often take intraoral images in daily clinical treatment, only approximately 35,000 intraoral images could have been stored between 2008 and 2019. The original PGGAN was trained with the CelebA dataset, which consists of approximately 200,000 face images12, and has achieved significant performance. Thus, our results may not utilize the maximum efficiency of PGGAN. In addition, our datasets contained a relatively higher number of images that showed healthy teeth than that of diseases, and there was also a tendency in PGGAN to generate healthy images compared to disease. If we were able to add images of diseases and adjust the class imbalance, we could have improved the performance of PGGAN. However, evaluators did not know the class imbalance of the datasets, and it is not affect the discrimination of images by dentists.
When dentists try to take intraoral images, cheek retractors and intraoral mirrors are placed in the mouth, and the camera axis and patient’s head should be parallel13. However, since most children cannot stay still and cannot open their mouth enough for an intraoral mirror to be inserted, it is difficult to obtain unified intraoral images. In addition, it is impossible to correct inappropriate intraoral images due to perspective and distortion, even if photo editing software is used. For these reasons, a large-scale unified and class-balanced public dental dataset needs to be constructed to enable the clinical application of deep learning with meaningful performance.
In order to challenge the generation of 1024 × 1024 with higher quality than PGGAN, another generative deep learning method is needed. For example, StyleGAN or StyleGAN2 can generate better images than PGGAN14,15. However, these new generative networks require a large amount of machine power. It has been reported that the training time of 1024 × 1024 resolution with StyleGAN is approximately one week, and that of StyleGAN2 is 9 days on NVIDIA DGX-1 with 8 Tesla V100 GPUs. If we try to perform these networks on our one TITAN RTX, it is estimated that the training time takes more than two or three months. On the other hand, there are few differences between 512 × 512 and 1024 × 1024 resolutions in dental diagnosis or examination, because dental conditions such as tooth shape, tooth color, tooth arrangement, caries, and metal crowns can be recognized with 512 × 512 resolution images. In addition, the training time of 512 × 512 resolution with PGGAN is approximately 8 days on TITAN RTX, which is a more acceptable time than StyleGAN. It is considered that PGGAN has sufficient ability to generate 512 × 512 or lower resolution intraoral images with reasonable machine power and time compared with new generative methods that require a large amount of computational resources.
It is critical to perform experiments that require domain-specific knowledge; however, the number of specialists is generally small and limited compared to general dentists. The 12 pediatric dentists in this study were almost all the pediatric dentists in our hospital, and it was almost the limit that cannot be increased further. Therefore, it is important to know in advance the number of specialists required for further studies. The number of participants that satisfied the statistical power of 0.9, was calculated to be 5.66 with our Cohen’s f (Table 2)10. If at least six specialists are recruited, it is possible that an experiment similar to our study can be performed in other medical fields.
Future direction and applications
There is a possibility that many types of images can be generated by changing the value of the latent vector. For example, image morphing can be performed by exploring the latent space16. In our study, morphing of intraoral images can be achieved by linearly interpolating the latent vectors that generate images of the primary dentition, mixed dentition, and permanent dentition (Fig. 6). It seems that images change transitionally between one end and the other as if the color changes with gradation. If arbitrary images can be generated with various types of images, PGGAN is a useful tool for dental education or for explaining materials to patients. Supplementary data showing intraoral image-generation movies can be found in the online version.
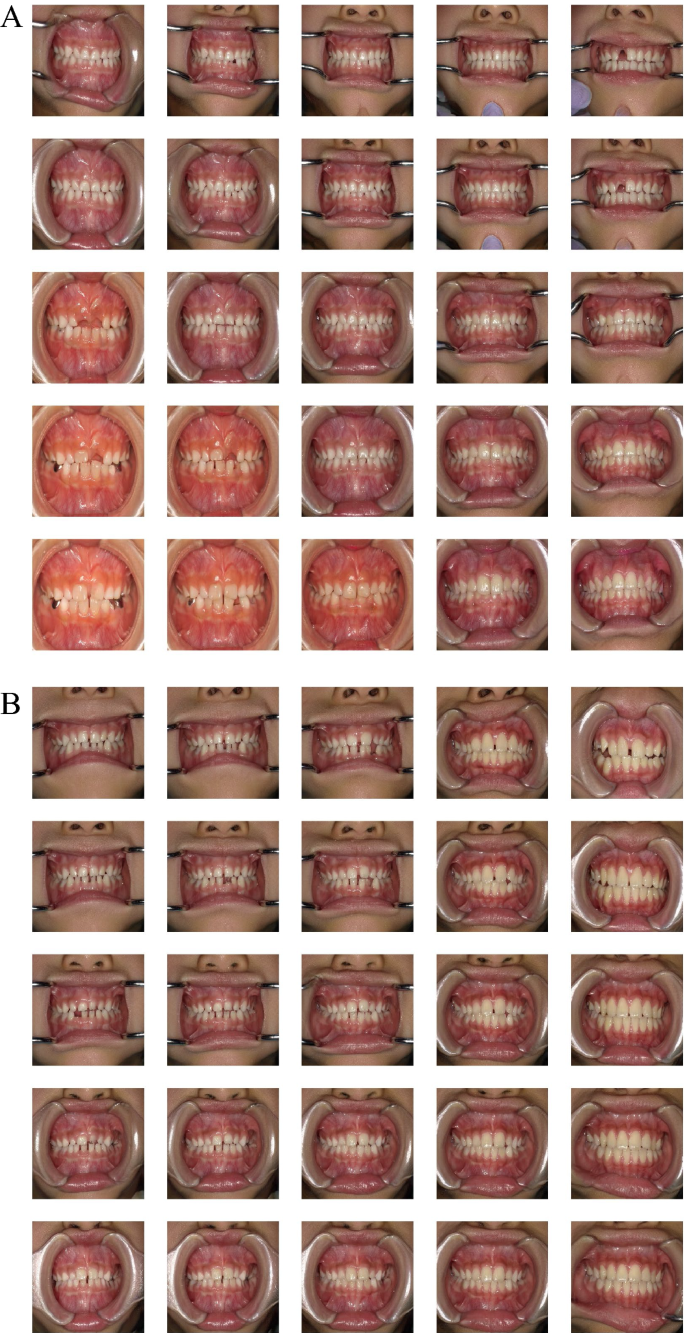
An example of exploring latent space. The images generated by PGGAN are gradually changing between one end and the other, from primary dentition to permanent dentition. One Supplementary Movie which shows intraoral image generation movie associated with this figure can be found in the online version.
Another advantage of using generated intraoral images is their ease of use. Because the images can be generated not to include private information, researchers or educators do not need to take care of individual information and can feel free to use the generated intraoral images.
In this study, we used the latent vector as input data for the PGGAN to generate images. If the PGGAN architecture is modified and the real images can be used for input data instead of the latent vector, such as pix2pix17 or CycleGAN18, there is a possibility that intraoral images of the future can be generated and predicted from current intraoral images. If children could be informed that their future teeth alignment is likely to be bad, early orthodontic treatment could be recommended, which would save money and time. Also, if intraoral images of the future could be generated that show the differences between getting treatment and not getting it, dentists would be better able to recommend dental treatment to their patients. Patients could be motivated to think about their oral health and be encouraged to brush their teeth carefully at home by being shown images of a future with periodontitis or tooth loss caused by not taking care of their teeth.
Another way of applying PGGAN is to use the generated images for data augmentation in deep learning. When we perform a deep learning project, many medical images for training data are required to achieve good performance. It is said that a small amount of training data can easily lead to overfitting in the deep learning model, so data augmentation of training images, such as translation, rotation, zoom, and contrast, is commonly used to reduce overfitting6. In addition to such data augmentation, it is expected that the generated realistic and varied images are used for deep learning as a method of data augmentation. It has been reported that the accuracy of medical image recognition based on deep learning has been improved by adding artificial images generated by GAN to the training data19,20,21. In the future, our findings may contribute to improving the performance of deep learning related to intraoral images by using generated images, since generated images are so realistic that pediatric dentists cannot distinguish which image is real or generated.